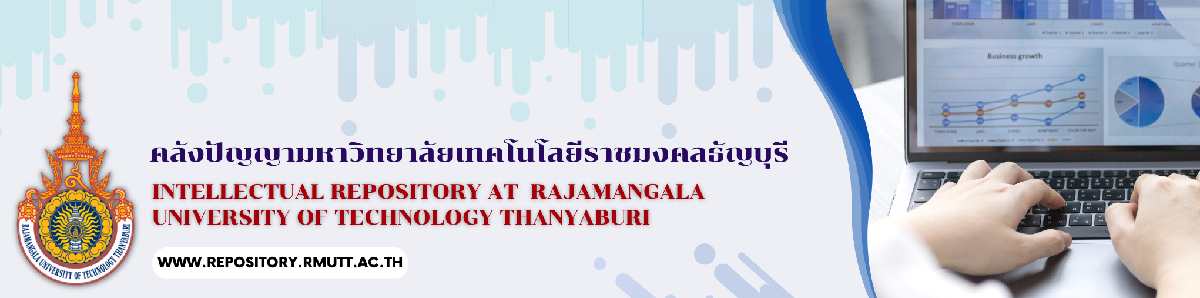
Please use this identifier to cite or link to this item:
http://www.repository.rmutt.ac.th/xmlui/handle/123456789/398
Full metadata record
DC Field | Value | Language |
---|---|---|
dc.contributor.author | Pattamavorakun, Suwat | |
dc.contributor.author | Pattamavorakun, Suwarin | |
dc.contributor.editor | Yang, Q | |
dc.contributor.editor | Webb, G | |
dc.date.accessioned | 2012-02-29T04:16:49Z | |
dc.date.accessioned | 2020-09-24T04:56:42Z | - |
dc.date.available | 2012-02-29T04:16:49Z | |
dc.date.available | 2020-09-24T04:56:42Z | - |
dc.date.issued | 2006 | |
dc.identifier.isbn | 3-540-36667-9 | |
dc.identifier.issn | 0302-9743 | |
dc.identifier.uri | http://www.repository.rmutt.ac.th/dspace/handle/123456789/398 | - |
dc.description.abstract | Recurrent Neural Networks (RNNs) are one in which self-loops and backward weight connections between neurons are allowed. As a result of these network characteristics, recurrent networks can address temporal behaviors which not possible in feedforward neural networks, such as their behavior in the limit reaches a steady state (fixed point), an oscillation (limit cycle),. and an a periodic instability (choas). Since RNNs have been increasingly applied to many dynamic system applications, there have been extensive efforts to develop a variety of architectures and training algorithms concerning on the enhancement of dynamic system characteristics. This work focuses on comparison of the selected and proposed training algorithms for RNNs. To evaluate the performance of the algorithms in the daily stock price forecasting in terms of efficiency index and computational time. A simple analysis on the complexity of RNNs was also carried out. It is noted that when comparing the speed of the algorithm, two components to be taken into account : the computation complexity and the space complexity. | en_US |
dc.language.iso | en | en_US |
dc.publisher | SPRINGER-VERLAG BERLIN | en_US |
dc.subject | Computer Science | en_US |
dc.title | A comparison of selected training algorithms for recurrent neural network | en_US |
dc.type | Article | en_US |
Appears in Collections: | บทความ (Article) |
Files in This Item:
File | Description | Size | Format | |
---|---|---|---|---|
A comparison of selected training algorithms for recurrent neural network.pdf | A comparison of selected training algorithms for recurrent neural network | 80.16 kB | Adobe PDF | View/Open |
Items in DSpace are protected by copyright, with all rights reserved, unless otherwise indicated.